Evaluation of Accuracy and Completeness of Electronic Dental Records in a Dental School Setting
Abstract
Background:
High-quality data in Electronic Dental Records (EDR) is essential for dental schools to provide high-quality patient care, improve dental students’ professionalism, and support a platform for research. Therefore, ensuring data quality in EDRs is extremely important.
Objective:
To perform a quality appraisal of EDRs by evaluating their accuracy and completeness.
Methods:
This was a Cross-sectional Observational Study conducted over four consecutive years in a dental school setting. Manual chart reviews were performed on an annual basis. EDR data were audited for accuracy and completeness. Accuracy was evaluated by comparing the entered data with an external source, where possible. An EDR data field was considered complete if it was not missing.
Results:
A total of 1,720 de-identified chart reviews were studied. The accuracy of the data to identify the patient was 93%. The completeness of the essential components of EDRs was 48-94%. Completeness was highest for documenting the patient’s chief complaint (94%) and the lowest for recall plan (48%). Completeness of data documenting social and behavioral determinants of health in EDRs was 36-77%, with the highest proportion of completeness in this domain being for oral hygiene habits, smoking habits, and social history.
Conclusion:
The quality appraisal of EDRs varied according to the data field. Understanding patterns of accuracy and completeness in EDRs will guide training and quality enforcement activities.
1. INTRODUCTION
The use of Electronic Health Records (EHRs) is considered one of the key advances in information technology. Eighty-one percent of US hospitals have been reported to use an EHR system [1], while in the Saudi Arabian capital, Riyadh, 50% of hospitals had fully functioning EHR systems, 36% had EHR systems in development, and 14% were still using paper-based records [2]. EHR systems specifically designed for dental practice are referred to as Electronic Dental Records (EDRs). In recent studies, 52% of US dental practices had adopted EDR systems [3], 52.8% of Brazilian dental offices used EDRs [4], while Scandinavian dental practitioners had 91.7% uptake of EDRs [5]. In Saudi Arabia, Almainman et al. studied the implementation experience of EDRs through interviews with stakeholders and found that staff satisfaction with EDRs was neutral at Saudi National Guard Health Affairs hospitals [6]. While EDRs overcame the problem of missing files in paper-based systems, the authors identified challenges in EDR use such as staff resistance to implementation and a lack of clear management change strategy [6].
There is now very good evidence that implementing EHRs is highly beneficial. EHRs improve documentation quality and reduce medical errors [7-10], the reporting of relevant clinical information increased 1.3-fold when electronic records were compared with a paper format [11], and there is less missing data in EHR systems [12]. The evolution of EHRs has also driven advances in technological healthcare delivery [13]. High-quality data in EDRs in dental schools has been shown to facilitate high-quality patient care, improve dental students’ professionalism, and provide a platform for research data [14]. As a result, there are increasing numbers of research papers on the use of EHRs as a data resource [15], emphasizing the importance and value of quality data in EHRs.
In order to assess data quality in EHRs, the National Institutes of Health (NIH) Health Care Systems Research Collaboratory suggested focusing on two characteristics, consistency and completeness. Consistency of data quality was categorized as either external or internal, where external consistency is equivalent to accuracy assessment and defined as “a comparison to an external source of information that is independent” [16]. External data consistency is also referred to as “data accuracy” or “data validity” [14], commonly via chart reviews. Internal consistency includes “programmed logic checks for procedure dates occurring after a death date or comparison of coded values between comparable sites in a multicentre study” [16]. This latter parameter could be improved by standardizing dental terminology across the dental profession [14, 17]. One success story in this regard is the Consortium of Oral Health Research and Informatics (COHRI), where participating dental schools created standardized data capture forms to form the “BigMouth” multi-institutional dental data repository [18]. With respect to EHR completeness, Weiskopf et al. reported variability in the proportion of complete records based on the definition used when comparing four definitions: documentation, breadth, density, and predictive completeness [19]. Since EHR completeness is contextual, they recommended that data consumers should themselves define what would be considered a complete record and address limitations of the chosen definition [19]. In light of this, the objective of this study was to perform a quality appraisal of EDRs by evaluating their accuracy and completeness in a dental school setting over four consecutive years.
2. METHODS
As a part of the quality assurance system at the University Dental Hospital, manual chart reviews were performed on an annual basis for quality improvement of EDRs. Eligibility criteria for inclusion of the EDR was an “active” file status. The American Dental Association (ADA) defines an active file as “the records of patients currently having their dental care provided by the practice”, while an inactive file is defined as “those patients who have not returned for 24 months” [20]. For chart review, an active file was defined as an EDR of a patient who had their most recent dental visit during that academic year.
Chart reviews were performed annually from 2016 to 2019. EDR data fields evaluated for quality appraisal were chosen based on Cruz and Rattan’s suggested essential components of dental records: data to identify the patient, medical history, dental history, extra-oral examination results, intra-oral examination results, charting of the teeth, periodontal charting and Basic Periodontal Examination (BPE) readings, radiographic examination, treatment plan developed, and recall period once treatment was completed [21]. Documentation of the patients’ chief complaint was added. In addition, data regarding social and behavioral determinants of health as described by the World Health Organization (WHO) were audited [22]. These included social history, employment status, housing information, patients’ level of education, barriers to access to oral health care (such as distance and transportation), oral hygiene habits (brushing and flossing), and smoking history.
Accuracy (or external consistency) was evaluated when possible by comparing the entered data with an external source. An EDR was considered complete if there were no missing data by adopting Liaw et al.’s definition of completeness [23]. A strict definition of completeness was adopted when evaluating the data field to ensure the proper use of the EDR system, meaning that if the information was present somewhere as free text in the EDR system but not in the designated data field, it was considered missing.
Statistical analyses were performed using IBM SPSS Statistics version 24.0 (2016, IBM Corp., Armonk, NY, USA). Descriptive summary statistics, including frequencies and percentages, were calculated from the de-identified chart review dataset.
3. RESULTS
A total of 1,720 de-identified chart reviews were used in this cross-sectional observational study. The accuracy of the data to identify the patient was determined by comparing the EDR entry to the patient’s ID. When it matched, the EDR data field was audited as accurate. Data accuracy for identifying the patient was 93%. Common reasons for inaccuracies included the missing middle name and misentered day or month in the date of birth field. The accuracy of the medical alert was determined by comparing the medical alert tab with the entry in the medical history and progress notes sections. However, the medical condition was for the vast majority documented in the progress notes of the initial examination visit and was not always translated to the medical alert tab (Fig. 1).
The proportion of completeness of the essential components of dental records ranged from 48% to 94% (Fig. 2).
Chief complaint, data to identify the patient, extra- and intraoral examination, and radiographic examination of EDR data fields were complete in ≥ 85% of cases. The recall period had a completeness of 48%. Completeness of social and behavioural determinants in EDRs ranged from 36% to 77% (Fig. 3), with the highest completeness for documenting oral hygiene habits, smoking habits, and social history. Interestingly, smoking habits were documented in 77% of cases. The lowest level of completeness was for housing information (33%).
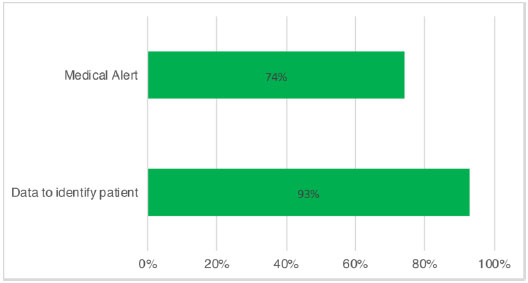
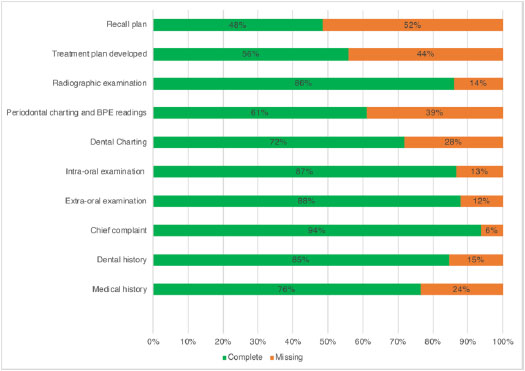
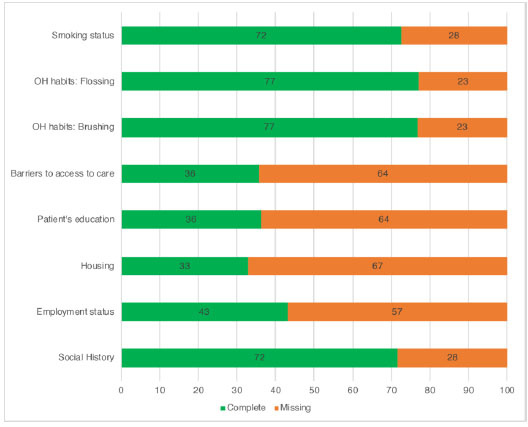
4. DISCUSSION
The results of this study contribute to our understanding of data accuracy and completeness in EDRs, particularly in the dental school setting. This setting is particularly important, as EDRs are considered a rich resource for research. For instance, a recent study relied on EDRs of a US dental school to evaluate attributes related to dental implant failures [15], while a Swedish study used EDRs to evaluate the association between demographic factors and dental health in children and adolescents [24].
We performed manual chart review rather than computerized surveillance, which might have been more time-consuming. However, manual chart review remains widely used and has been shown to be more accurate than computerized surveillance [25]. This annual chart review was part of annual quality assurance monitoring, where EHRs have been shown to support quality management by promoting better clinical outcomes, managerial monitoring, cost-effectiveness, political goals, and strategies [26].
This study focused on structured data types rather than on unstructured data, as chart review was geared towards checking on the appropriate use of designated data fields. The majority of published literature in this area has similarly focused on structured data [27]. We used two quality measures: accuracy and completeness, where completeness is the most commonly used measure for assessing EHR quality (64%) [27]. The average completeness for all the audited data fields was 77%. Although this may seem relatively low, it is consistent with the proportion of quality documentation of contrast agent allergies in EHRs (71.8%) [28]. Smoking status was documented in 77% of the audited EDRs, which is much higher than that previously reported for EHRs (30%) [29]. Consistent with this, Patel et al. reported that EDRs are a more valuable resource than EHRs to obtain data regarding smoking behaviour [30].
Finally, our results suggest that the lowest completeness was for the recall plan data field. The reporting of recall plan completeness is mostly underestimated in this study, as this field was audited regardless of the patient’s treatment phase. Moreover, recall period was often found in the progress notes section of the phase-I evaluation visit but was marked as “missing” in our study when not reported in the assigned data field “recall plan”. Improving documentation in the designated data field can only be achieved by properly training users to use the data fields and to understand the importance of such practice. Although suboptimal levels of completeness might be of concern, our study findings are not surprising considering that there is evidence of a poor agreement between patient self-reporting and EHR documentation (with more ocular symptoms reported on the patient self-reporting forms in one study [31]). Dental charting, periodontal charting, and developing a treatment plan completeness were between 56 and 72%, which may have been affected by the fact that patients that present only for an emergency dental visit do not receive complete charting (unless they also attend for a routine dental appointment at a later date). Carsley et al. documented the differences in data completeness between routine and emergency visits and found that completeness of body mass index data was higher at well-child visits (89.9%) than at sick visits (66.2%) [32].
The strengths of this study are in adopting strict definitions for quality appraisal and having a large sample size. However, this study has several limitations. EDR data were from a single dental school setting, which may limit this study’s generalizability. Also, the study might have overestimated the proportion of incomplete records, as an EDR data field was considered incomplete when it was not documented under the designated data field, even if the information was documented somewhere as free text in other fields. The reason for adopting this strict definition of quality appraisal was to ensure proper use of the EDR system rather than relying on time-consuming searches of unstructured free-text data. Future directions would be to compare the quality appraisal of EDRs in different dental settings, users’ experiences, and explore factors that may affect EDR quality. A better understanding of the current status of EDR data quality and the modifying factors could help to tailor directed training for EDR users. Ultimately, this will improve data quality and empower the use of EDR for any purpose.
CONCLUSION
In conclusion, quality appraisal of EDRs in terms of accuracy and completeness varied according to the data field. Data was 93% accurate for identifying the patient. Completeness of essential components of dental records ranged from 48% to 94%. Patients’ chief complaints had the highest proportion of completeness, while the recall plan had the lowest proportion of completeness. Completeness of social and behavioural determinants of health ranged from 33% to 77%, with the highest proportion for smoking status, oral hygiene habits, and social history. Understanding patterns of accuracy and completeness in EDRs will guide training and quality enforcement activities.
ETHICAL APPROVAL AND CONSENT TO PARTICIPATE
King Abdulaziz University Faculty of Dentistry research ethics committee approved the study protocol (No. 114-07-19), Jeddah, Saudi Arabia.
HUMAN AND ANIMAL RIGHTS
No Animals were used in this research. All human research procedures followed were in accordance with the ethical standards of the committee responsible for human experimentation (institutional and national), and with the Helsinki Declaration of 1975, as revised in 2013.
CONSENT FOR PUBLICATION
Signed informed consent was obtained from each patient having an electronic dental record.
AVAILABILITY OF DATA AND MATERIALS
The authors confirm that the data supporting the findings of this study are available within the article.
FUNDING
None.
CONFLICTS OF INTEREST
The author declares no conflict of interest, financial or otherwise.
ACKNOWLEDGEMENTS
The author would like to thank Ghadi Y. Al-Malki for her assistance in data management.