All published articles of this journal are available on ScienceDirect.
The Role of Artificial Intelligence in Transforming Dental Public Health: Current Applications, Ethical Considerations, and Future Directions
Abstract
Background
Artificial Intelligence (AI) and its subset, Machine Learning (ML), have emerged as pivotal tools in dental public health by enhancing diagnostic accuracy, optimizing resource allocation, and personalizing patient care. AI offers significant opportunities, particularly in predictive analytics, personalized interventions, and expanding access to underserved areas.
Objective
This review presents a broad evaluation of AI’s applications in dental public health, emphasizing their roles in diagnostics, discussing ethical considerations, and outlining future directions to enhance public health practice and policy.
Methods
We conducted a literature review of published articles from major databases (e.g., PubMed, Scopus, Web of Science) between 2015 and 2024. We used relevant search terms (e.g., “Artificial Intelligence,” “Machine Learning,” “Dental Public Health,” “Ethical Considerations,” “Predictive Analytics”). We applied inclusion and exclusion criteria based on relevance to diagnostics, epidemiological data analysis, health promotion, and ethical/legal frameworks.
Results
The findings indicate that AI technologies enhance diagnostics (e.g., interpreting dental radiographs), support predictive analytics for disease monitoring, and enable targeted health interventions. However, significant challenges include data privacy risks, algorithmic biases, and compatibility with existing infrastructures. Ethical considerations, such as transparency, accountability, and equitable access are essential for responsible AI integration.
Conclusion
AI holds the potential to revolutionize dental public health by improving patient outcomes, especially in underserved populations. Further interdisciplinary research, clear policy frameworks, and strong data protection measures are required to ensure the ethical and effective deployment of these technologies. Future investigations should also foster interprofessional collaboration to maximize the benefits of AI in dental public health.
1. INTRODUCTION
Conventional public health approaches in dentistry have historically relied on manual diagnostics, analog radiographic interpretation, and population-level screening methods that can be labor-intensive and prone to human error. These traditional methods may lead to delays in diagnosis and create inefficiencies in resource allocation. As a result, underserved communities may face ongoing disparities in oral health outcomes [1].
In this context, Artificial Intelligence (AI) and, specifically, Machine Learning (ML) have emerged as a transformative force. These technologies are reshaping conventional approaches by enhancing diagnostic accuracy, optimizing resource allocation, and enabling personalized patient care. AI’s ability to analyze large datasets quickly can also help identify trends and risk factors at the population level, promoting more targeted interventions. Despite these benefits, caution is warranted. Ethical considerations including data privacy, biases in algorithms, and equitable access require ongoing attention to ensure that AI tools serve the greater public interest.
To address these points, this manuscript aims to (1) describe the transformative role of AI and ML in dental public health, (2) examine their current applications, (3) discuss ethical implications, and (4) propose future directions. By evaluating both the potential and limitations of these technologies, this review strives to equip readers with actionable strategies to responsibly deploy AI in ways that enhance patient and population outcomes.
1.1. Introduction to Artificial Intelligence and Machine Learning
AI and ML are rapidly evolving technologies with significant potential in healthcare. AI refers to machine-based systems capable of performing tasks typically requiring human intelligence. In contrast, ML is a subset of AI that focuses on algorithms that learn from data and improve over time. Together, these technologies support tasks like complex diagnostics, treatment planning, and public health surveillance [2].
1.2. Relevance of AI and ML in Dental Public Health
AI and ML can profoundly impact dental public health by enhancing disease diagnosis, personalizing patient care, and optimizing resource allocation. For example, AI has been utilized to interpret dental radiographs with high accuracy, aiding in diagnosing conditions such as caries and impacted teeth, thus streamlining screening and intervention programs [3]. Furthermore, AI applications can analyze large datasets to identify trends and predict outbreaks, helping shape data-driven public health responses [4].
2. METHODS
A broad-based literature review was conducted to identify peer-reviewed articles focusing on AI applications in dental public health between 2015 and 2024. The databases searched included PubMed, Scopus, and Web of Science. Key search terms were “Artificial Intelligence,” “Machine Learning,” “Dental Public Health,” “Ethical Considerations,” “Predictive Analytics,” and “Personalized Interventions.” Inclusion criteria required that articles address AI or ML in a dental public health context, including but not limited to diagnostics, epidemiological analysis, data privacy, or policy implications. Exclusion criteria omitted studies unrelated to dentistry or lacking sufficient methodological detail.
3. RESULTS
3.1. Current Applications of AI and ML in Dental Public Health
3.1.1. Automated Diagnostics
AI has significantly advanced automated diagnostics in dental public health. For instance, deep learning models like BDU-Net (an architecture designed for precise segmentation tasks) have been developed to enhance diagnostic accuracy from panoramic radiographs. These models demonstrate high sensitivity and specificity in detecting various dental issues, such as caries and impacted teeth, thereby enabling rapid identification of disease—an essential component of timely public health interventions [3].
3.1.2. Epidemiological Data Analysis
Machine learning algorithms are extensively utilized in analyzing large datasets to identify patterns that might not be evident through traditional epidemiological methods. In dental public health, AI models can process vast amounts of dental health data to forecast trends and potential outbreaks, helping public health officials plan and execute health programs more effectively [2].
3.1.3. Health Promotion and Disease Prevention
AI plays a pivotal role in health promotion and disease prevention within dental public health. By analyzing demographic and health data, AI systems can identify at-risk populations and tailor preventive measures accordingly. These systems enable personalized public health messages and interventions, which can be directed at specific groups based on their risk profiles, enhancing the efficiency and effectiveness of public health campaigns [5, 6].
3.2. Technological Framework and Methodologies in Dental Public Health
3.2.1. Data Collection
Effective AI applications in dental public health rely heavily on robust data collection systems. These systems gather various health data, including dental health records, epidemiological studies, and population health metrics. Integrating AI/ML technologies leverages large data repositories to analyze and predict health outcomes, thus enhancing disease surveillance and management capabilities [7].
3.2.2. Machine Learning Models
Machine learning models form the backbone of AI applications in dental public health by employing techniques such as deep learning to process and analyze large datasets. These models categorize and predict dental health outcomes with high accuracy, which is critical for strategic resource allocation and the planning of public health interventions. Neural networks and decision trees are commonly used in this domain [8].
3.2.3. Integration with Existing Systems
Integrating AI technologies with existing dental public health systems poses significant challenges, including compatibility with current Information Technology (IT) infrastructures and the need for comprehensive training for health professionals. Despite these challenges, AI can enhance the overall functionality and efficiency of service delivery. Successful integration strategies focus on designing AI systems with a clear understanding of existing workflows and user needs in public health settings [4].
Moreover, broader healthcare transformations predicted by 2024 underscore how AI may reshape public health approaches on a large scale [9]. Recent analyses also point to generative AI solutions that can enable faster data integration, better disease modeling, and more targeted public health interventions [10].
3.3. Challenges and Limitations of AI and ML in Dental Public Health
3.3.1. Data Privacy and Security
One of the most significant challenges in implementing AI in dental public health is ensuring data privacy and security. AI systems typically process large volumes of private health data, exposing them to potential breaches if not properly protected. Robust security measures and compliance with legal and ethical standards are necessary to protect patient information [11]. According to the World Health Organization (WHO), data governance frameworks must consider cross-border data transfers and encryption standards that safeguard patient records [7].
3.3.2. Interpretability and Transparency
AI models in healthcare often suffer from a lack of interpretability, commonly called the “black box” problem. This issue arises because AI algorithms, particularly those involving complex machine learning models, can be difficult to understand. This lack of transparency can lead to skepticism and lower trust among healthcare providers and patients, potentially hindering the adoption of AI technologies [4].
3.3.3. Infrastructure and Integration Challenges
Challenges integrating AI technologies into existing dental public health systems often face significant obstacles, including inadequate infrastructure and the complexity of merging AI tools with regulatory systems. Data siloing, system interoperability, and policy misalignment remain core barriers to seamless adoption [11].
3.3.4. Ethical Concerns
Applying AI in dental public health raises several ethical concerns, including potential biases in AI algorithms, which can perpetuate existing inequalities. For example, AI systems might produce skewed results if trained on datasets that underrepresent certain populations. Addressing these biases is crucial for ensuring equitable healthcare outcomes and maintaining trust in AI applications [4].
3.3.5. Skills Gap
The successful deployment of AI in dental public health requires a workforce skilled in AI technologies, data science, and their applications in healthcare. Currently, there is a shortage of professionals with these specialized skills, limiting its effective adoption [11].
3.3.6. Cost
The financial barriers associated with implementing AI are significant. Hardware, software licenses, and specialized training contribute to high upfront and maintenance costs, making AI less accessible for budget-constrained public health organizations [11].
These challenges highlight the complexities of integrating AI into dental public health. Addressing these issues requires concerted efforts to improve AI transparency, enhance data security, ensure ethical deployment, and invest in workforce training and infrastructure.
3.4. Case Studies and Current Research in AI and ML in Dental Public Health
3.4.1. Successful Implementations: AI in Periodontal Disease Diagnosis
A noteworthy case of a successful AI implementation in dental public health involves the use of deep convolutional neural networks (CNNs) to diagnose periodontal conditions. These AI systems analyze dental images with high precision, thereby enhancing the detection and classification of periodontal diseases. For example, CNNs have been employed to evaluate the severity of periodontal compromise and accurately predict the prognosis of Periodontally Compromised Teeth (PCT). This AI application not only improves diagnostic accuracy but also assists in better treatment planning by predicting potential outcomes, allowing for more effective management of periodontal diseases [12].
The utilization of CNNs represents a significant advancement over traditional diagnostic methods, which often rely heavily on the subjective judgment of clinicians. By incorporating AI, clinicians can now perform a more objective analysis of dental images, leading to increased consistency and reliability. This technology has been shown to improve the accuracy of diagnosing specific conditions, such as differentiating between premolars and molars in periodontal assessments, which can present challenges due to their anatomical differences [12].
3.4.2. Predictive Analytics: Enhancing Diagnostic Accuracy with AI
Predictive analytics is revolutionizing dental public health by enhancing the ability to forecast health outcomes and tailor interventions. These AI-driven models analyze large data, detecting patterns that help predict the risk of dental diseases, such as caries or periodontal diseases before they manifest visibly. By employing advanced AI techniques, these models can identify carious lesions and other pathological changes with greater accuracy at earlier stages, thereby facilitating earlier interventions and improving patient outcomes [2, 3].
Implementing AI and ML from the ground up has also been explored in practical case studies, highlighting the operational steps needed to harness predictive analytics effectively [13]. Furthermore, advanced AI solutions in clinical settings illustrate real time analytics for risk stratification and decision support [14], while surveys in healthcare predictive analytics identify key machine learning and deep learning techniques that could be readily adapted for dental public health [15].
3.4.3. Personalized Public Health Interventions
The integration of AI into dental public health is paving the way for interventions tailored to individual risk factors. By leveraging machine learning algorithms, public health professionals can develop customized prevention programs that consider demographic, lifestyle, or genetic data, such as genetic predispositions and lifestyle factors. This personalization helps in crafting preventative strategies and treatments, ultimately bolstering patient engagement and adherence [16, 17].
3.4.4. Expansion into Underserved (Low-Resource) Settings
AI technologies hold promise for expanding dental public health services into underserved settings. These technologies can optimize resource allocation, improve diagnostics as well as treatment planning via tele-dentistry, and support local healthcare providers with decision-making tools that require minimal infrastructure. AI can facilitate broader access to dental care in communities where traditional healthcare infrastructure may be lacking, thus extending the impact of dental public health initiatives [18, 19].
These advancements highlight the transformative potential of AI in dental public health, indicating a shift towards proactive, personalized, and accessible dental care solutions. Ongoing development in AI-driven approaches promises to enhance oral health outcomes further and streamline service delivery.
Through various computer vision techniques and machine learning models, AI has been employed to enhance dental disease diagnosis, thereby improving patient outcomes [16]. The role of neural networks in dental care, particularly in the detection and classification of dental caries and restorations, is another critical area. These approaches often surpass traditional methods in terms of both precision and efficiency [16]. Moreover, insights from the Fédération Dentaire Internationale (FDI) highlight the global perspective on AI integration, urging the adoption of transparent, equitable, and scientifically valid practices [8]. Additionally, integrative multi-omics data analysis is emerging as a crucial element in personalizing interventions further, bridging clinical data with broader population-level insights [20].
3.5. Ethical Considerations in AI for Dental Public Health
3.5.1. Ethical Frameworks and Guidelines
The ethical integration of AI into dental public health necessitates adherence to established ethical frameworks that emphasize transparency, accountability, and patient-centric values. The World Health Organization (WHO) enumerates six core principles for ethical AI in healthcare: (1) protecting autonomy, (2) promoting well-being and safety, (3) ensuring transparency and intelligibility, (4) fostering responsibility and accountability, (5) ensuring inclusiveness and equity, and (6) promoting sustainable and responsive AI systems [7].
3.5.2. Informed Consent and Data Privacy
Informed consent is a critical aspect of deploying AI in healthcare. Patients must be adequately informed about how their data will be used, the benefits, and the risks involved. Additionally, stringent measures must be taken to protect private patient data and prevent breaches that could compromise patient confidentiality and privacy [5, 6].
3.5.3. Bias and Discrimination
AI systems must be designed to mitigate bias that can lead to discrimination in patient care. This includes ensuring that AI algorithms are trained on appropriately representative datasets to avoid perpetuating existing health disparities. Ensuring fairness in AI applications is essential to prevent biases related to age, ethnicity, gender, or socioeconomic status from influencing the outcomes of AI-assisted decisions [5, 21].
3.5.4. Transparency and Accountability
Transparency in AI operations allows patients and providers to understand how AI systems make decisions. This transparency is vital for building trust and for clinicians to feel confident about integrating AI tools into their practice. Accountability mechanisms should also be in place to address any adverse outcomes associated with AI interventions, ensuring that these technologies are used responsibly [6, 21].
3.5.5. Interoperability and Integration
For AI to be effectively integrated into existing dental practice workflows, it must be interoperable with current systems. Standards and guidelines help achieve this integration, ensuring that AI tools can communicate seamlessly with other healthcare technologies, thus minimizing disruptions to clinical workflows [6].
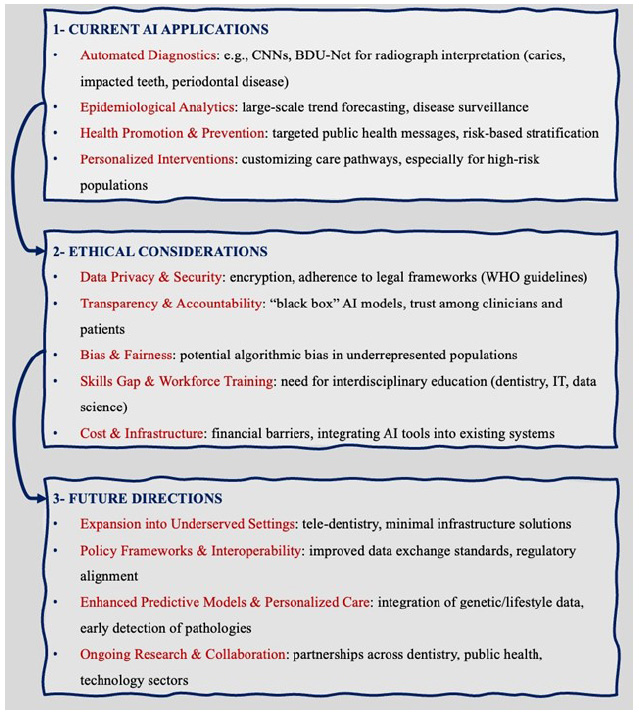
A structured view of the main themes in AI and dental public health.
3.5.6. Continuous Learning and Adaptation
As AI technology evolves, ongoing education and training for healthcare providers are imperative. This ensures that dental professionals remain knowledgeable about the latest developments and can use AI tools effectively and ethically. Moreover, AI systems themselves should be capable of adapting to new data and changing healthcare landscapes to continuously provide optimal support in dental public health settings [5].
4. DISCUSSION
In light of the reviewed literature (Fig. 1), AI’s role in dental public health emerges as a mechanism to enhance diagnostic precision and resource allocation while simultaneously offering personalized patient interventions. However, the successful implementation of AI is contingent upon addressing ethical, infrastructural, and workforce challenges.
Studies focusing on automated diagnostic tools (e.g., CNNs, BDU-Net) underscore AI’s capability to detect pathologies earlier than human-based assessment alone, thus positioning AI as a complementary tool rather than a complete substitute for clinical expertise [3, 12]. Predictive models show promise for identifying high-risk populations and tailoring preventive campaigns accordingly [2, 16]. At the same time, data management and privacy stand out as crucial considerations; even minor breaches in data security can erode patient trust and stall progress [11].
Moreover, integrating AI into existing public health systems must involve robust planning to ensure compatibility with existing IT infrastructures and regulatory environments. Large-scale adoption is made more difficult by the personnel gap, which includes medical professionals who are trained in both machine learning and dentistry. Public institutions with constrained budgets may also struggle to prioritize AI-related expenditures, thereby widening the digital divide [8, 11].
Ethically, the literature highlights the need to mitigate biases in AI algorithms that might disadvantage vulnerable groups. Ensuring equitable access and maintaining transparency are thus non-negotiable for the responsible application of AI in dental public health [4, 5].
In summary, AI can act as a catalyst for innovation in dental public health, enabling more proactive and individualized patient care. Yet, collaborative policymaking, interdisciplinary research, and targeted investments in training and infrastructure are essential to ensure these technologies align with ethical norms and serve the broader societal good.
CONCLUSION
AI and ML technologies hold the potential to revolutionize dental public health by enhancing diagnostic accuracy, optimizing resource allocation, and delivering personalized patient care. However, responsible integration must prioritize privacy, equity, transparency, and interdisciplinary collaboration. With well-crafted policies and ongoing research, AI can significantly bolster oral health outcomes, particularly in underserved populations, and pave the way for a more efficient and equitable future in dental public health.
AUTHORS’ CONTRIBUTION
M.B.: Study conception and design, data collection, analysis and interpretation of results, and manuscript preparation.
LIST OF ABBREVIATIONS
AI | = Artificial Intelligence |
ML | = Machine Learning |
CNN | = Convolutional Neural Networks |
PCT | = Periodontally Compromised Teeth |
WHO | = World Health Organization |
FDI | = Fédération Dentaire Internationale (World Dental Federation) |
ACKNOWLEDGEMENTS
The authors would like to thank colleagues at the Department of Dental Public Health, King Abdulaziz University, for their guidance and support in the preparation of this review.