All published articles of this journal are available on ScienceDirect.
Uracil Biomarker for Pyrimidine Metabolism in OSCC: Leveraging AI and Machine Learning for Improved Diagnosis
1. INTRODUCTION
Oral Squamous Cell Carcinoma (OSCC) [1-3], especially Gingivobuccal OSCC (GB-OSCC), is a notably aggressive form of cancer characterized by its rapid advancement and tendency for metastasis. Recent developments in cancer biology have identified metabolic reprogramming as a crucial element in cancer survival and proliferation [4]. In particular, the metabolism of pyrimidines, with uracil as a key metabolite, has been recognized as a significant metabolic biomarker within the Tumor Microenvironment (TME) [5]. This article explores the role of uracil as a marker for pyrimidine metabolism in OSCC and discusses potential directions for integrating Artificial Intelligence (AI) and Machine Learning (ML) to improve early diagnosis and personalized cancer treatment.
1.1. Biomarkers and Pyrimidine Metabolism in OSCC
Uracil, a principal metabolite of pyrimidines, has been identified in more than 83% of OSCC tumor samples, underscoring its potential as a metabolic biomarker. Pyrimidine metabolism plays a crucial role within the TME, supporting tumor survival and aiding in immune system evasion [5]. Increased levels of uracil are strongly associated with lymph node metastasis, positioning it as an important prognostic indicator. Additionally, enzymes, such as CD39 and P2Y6, which are involved in the synthesis of uracil, have been found to be overexpressed in aggressive OSCC, presenting potential therapeutic targets for disrupting these metabolic pathways to impede tumor growth and dissemination [6].
The reprogramming of uracil metabolism within the TME plays a central role in tumor advancement, making it an attractive candidate for diagnosing and treating OSCC. Fig. (1) represents the pyrimidine metabolism pathway in the TME, emphasizing the energetic interaction between tumor and immune cells in regulating uracil levels [7]. Tumor cells elevate uracil concentration through the breakdown of pyrimidine nucleotides (uridine triphosphate → uridine diphosphate → uridine monophosphate), facilitated by the enzymatic actions of Ectonucleoside Triphosphate Diphosphohydrolases (ENTPD5 and ENTPD4) [8]. Furthermore, Uracil Phosphoribosyltransferase (UPRT) supports uracil salvage, reinforcing pyrimidine biosynthesis essential for the rapid proliferation of tumor cells [9]. This metabolic activity promotes not only tumor growth, but also immune evasion via P2Y6 receptor activation, which regulates UDP signalling and intracellular pathways [10].
Conversely, immune cells in the TME depend on CD39 and ENTPD4 enzymes to degrade extracellular ATP and UDP, resulting in adenosine production, a strong immunosuppressive molecule. Adenosine diminishes the functionality of cytotoxic T lymphocytes and Natural Killer (NK) cells, shielding tumor cells from immune responses [5]. This metabolic competition between tumor and immune cells creates a finely balanced TME, simultaneously driving tumor expansion and immunosuppressive conditions. Alterations in pyrimidine metabolism often signal OSCC progression, emphasizing its potential as a diagnostic and therapeutic focal point.
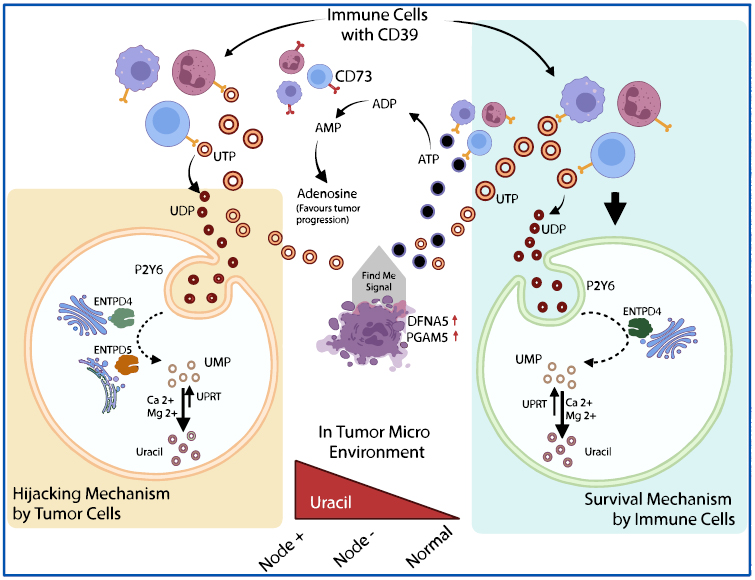
Schematic representation of pyrimidine metabolism in the tumor microenvironment, illustrating the distinct roles of tumor and immune cells. Available online under the terms of the Creative Commons Attribution Non-Commercial License 4.0.[5].
Cutting-edge multi-omics approaches, including metabolomics, transcriptomics, and proteomics, provide an integrated perspective on these metabolic shifts [11]. AI-enabled tools are vital for analysing complex data sets, revealing aberrations in uracil metabolism, predicting OSCC development, and pinpointing actionable biomarkers. For instance, AI algorithms applied to biofluid metabolomics can identify elevated uracil levels in saliva or plasma, enabling non-invasive and efficient OSCC detection [12]. Machine learning models further enhance diagnostic precision by correlating metabolic abnormalities with clinical and histopathological insights to forecast therapeutic outcomes [13].
Immunohistochemistry (IHC) offers complementary benefits by visually mapping the distribution of key metabolic enzymes, such as CD39, ENTPD4, and UPRT, within tumor and immune cells [5]. This technique quantitatively evaluates protein expression levels, linking these markers to tumor aggressiveness and patient prognosis. AI-powered Convolutional Neural Networks (CNNs) streamline the analysis of IHC images, allowing high-throughput and precise assessment of metabolic remodelling in OSCC [14]. RNA Sequencing (RNA-Seq) unravels gene expression profiles associated with pyrimidine metabolism and immune evasion strategies. When integrated with pathway enrichment analyses, these datasets can identify novel therapeutic targets, such as inhibitors of ENTPD5 or regulators of P2Y6 signalling. Such discoveries can fuel precision medicine initiatives, with AI accelerating the design and screening of small-molecule inhibitors aimed at these metabolic enzymes [15]. The uracil metabolism within the TME serves as a crucial intersection of tumor progression, immune modulation, and therapeutic exploration. Leveraging multi-omics frameworks combined with AI technologies facilitates the deconstruction of metabolic interactions, empowering early diagnosis, better prognostic models, and targeted treatment options for OSCC.
1.2. Role of AI and Machine Learning in Enhancing Cancer Therapy
Utilizing uracil as a biomarker for pyrimidine metabolism in OSCC shows great potential, especially when combined with AI and ML. Metabolomics analysis, using techniques, like Nuclear Magnetic Resonance (NMR) spectroscopy and Mass Spectrometry (MS), measures uracil levels in blood, saliva, or tumor tissues, revealing its role in OSCC and metabolic changes.
AI and ML techniques, including deep learning and Support Vector Machine (SVM), analyse complex metabolomics data to identify patterns in uracil expression. This enables early cancer detection and improved diagnostic accuracy. Predictive models using machine learning methods, such as random forest and logistic regression, can forecast cancer progression and survival rates based on uracil levels, offering precise prognostic information.
Integrating multi-omics data metabolomics, genomics, and transcriptomics provides a comprehensive view of cancer biology. AI models can correlate uracil levels with genetic mutations and gene expression, enhancing diagnosis and therapy development. Unsupervised learning techniques, like clustering algorithms, may discover new biomarkers by revealing novel metabolic patterns. AI-powered biosensors enable continuous, non-invasive monitoring of uracil levels, offering real-time insights into OSCC progression. Personalized treatment strategies, guided by AI analysis of uracil levels and genetic profiles, can tailor therapies to individual patients, optimizing treatment efficacy [16, 17].
1.3. Improving OSCC Diagnosis and Treatment with AI and NMR
Advancing the diagnosis and treatment of OSCC through technologies, like AI and NMR, opens new possibilities in cancer care. Targeting pyrimidine metabolism, particularly enzymes, like CD39 and ENTPD4, involved in uracil synthesis, presents a viable therapeutic approach. Inhibiting these enzymes, which are essential for tumor growth, disrupts key metabolic pathways vital for cancer cell survival. Additionally, blocking the P2Y6 receptor, responsible for the uptake of Uridine Diphosphate (UDP) for uracil synthesis, can starve cancer cells of crucial metabolites. This strategy may be especially effective in patients with high uracil levels, notably in node-positive OSCC cases.
Combining NMR, Immunohistochemistry (IHC), and RNA Sequencing (RNA-Seq) with AI and machine learning can significantly improve diagnostic accuracy and therapeutic precision in OSCC. Focusing on uracil as a biomarker of pyrimidine metabolism, NMR analysis provides detailed metabolic profiles, revealing specific metabolic signatures linked to OSCC. AI algorithms can classify these samples, identify biomarkers, like uracil, and assess metabolic pathways, thereby enhancing both diagnosis and treatment planning [18]. This underscores the pivotal roles of uracil metabolism in tumor advancement and immune regulation, and utilizing AI for OSCC detection and therapy. The study highlights the crucial roles of various factors in tumor metabolism and their intricate mechanisms within the TME. Elevated uracil levels (75%) act as a pivotal diagnostic marker for tumor progression, with AI-powered metabolomics facilitating non-invasive OSCC detection [19]. Adenosine production (65%) bolsters immune evasion, with AI models predicting levels of immune suppression [20]. Similarly, P2Y6 receptor activation (60%) promotes tumor survival via UDP signalling, as AI maps receptor expression for targeted therapeutics [21]. Targeting uracil metabolism (70%) disrupts pyrimidine biosynthesis, with AI-accelerated drug screening enhancing efficacy. Furthermore, tumor-immune pyrimidine competition (50%) uncovers dynamic TME interactions, decoded through deep learning-based multi-omics analyses [5, 22].
Expanding these insights, Immunohistochemistry (IHC) staining offers spatial and quantitative data on protein markers, like CD39 and CD73, which significantly influence tumor advancement. AI-driven Convolutional Neural Networks (CNNs) streamline image analysis, quantifying marker expression and linking levels to clinical prognoses. Concurrently, RNA Sequencing (RNA-Seq) unveils gene expression patterns that serve as biomarkers or therapeutic targets. By integrating expression data with pathway enrichment analyses, machine learning predicts clinical outcomes and identifies critical molecular pathways, illuminating OSCC progression and aiding precision medicine. Incorporating AI and machine learning with these methodologies could enhance the detection of metabolic biomarkers in GB-OSCC. AI excels in analysing NMR spectral data, accurately identifying metabolites, like uracil, and distinguishing tumor from normal tissue. This precision strengthens uracil detection and highlights its significance in GB-OSCC. Furthermore, AI enables the integration of data from NMR, IHC, and RNA-Seq, correlating uracil levels with gene and protein markers, such as CD39 and CD73, offering valuable insights into the relationships between these markers and disease progression [23].
AI-driven predictive models also reveal links between uracil expression and clinical features, like lymph node metastasis, informing personalized treatment strategies. Machine learning algorithms, such as clustering and classification, identify gene upregulation patterns, including PGAM5 and DFNA5, associated with OSCC pathogenesis. Lastly, AI uncovers functional relationships between metabolic pathways and tumor biology, demonstrating how CD39, CD73, and the P2Y6 receptor contribute to an immunosuppressive tumor microenvironment. These innovations are revolutionizing biomarker discovery, diagnostic accuracy, and prognosis in GB-OSCC [24, 25].
CONCLUSION
Integrating pyrimidine metabolism biomarkers, such as uracil, with AI-driven multi-omics approaches represents a breakthrough in addressing OSCC, particularly Gingivobuccal OSCC (GB-OSCC). Elevated uracil levels, a hallmark of disrupted pyrimidine metabolism, serve as critical diagnostic and prognostic markers linked to tumor progression, immune evasion, and lymph node metastasis. Enzymes, like CD39, ENTPD4, and UPRT, drive metabolic shifts in the TME, creating therapeutic opportunities by targeting pathways, like UDP-P2Y6 signalling. AI and ML significantly enhance biomarker discovery, diagnosis, and therapy. Advanced AI models, including convolutional neural networks for Immuno-histochemistry (IHC) analysis and ML frameworks for multi-omics data integration, facilitate precise evaluation of uracil metabolism. Techniques, like NMR spectroscopy, RNA sequencing, and metabolomics, combined with AI, improve non-invasive biomarker detection and enable personalized treatments. AI also accelerates drug discovery by identifying inhibitors for enzymes, such as ENTPD5, and receptors, like P2Y6. AI-driven insights into tumor-immune metabolic interactions highlight the potential of disrupting pyrimidine metabolism to inhibit tumor growth and immune suppression. Future efforts should focus on clinical validation, refining predictive models, and exploring combination therapies. This integration of AI with cancer biology offers a transformative pathway for improving OSCC diagnosis, prognosis, and treatment.
AUTHORS’ CONTRIBUTION
S.B. and D.G.: Study conception and design, data collection, and analysis and interpretation of results; S.B.: Drafting of the manuscript; D.N.: Resource allocation and validation; A.K.S.: Editing, reviewing, and supervision. All authors have reviewed the results and approved the final version of the manuscript.
LIST OF ABBREVIATIONS
TME | = Tumor Microenvironment |
OSCC | = Oral Squamous Cell Carcinoma |
ML | = Machine Learning |
CNNs | = Convolutional Neural Networks |
MS | = Mass Spectrometry |
NMR | = Nuclear Magnetic Resonance |
UPRT | = Uracil Phosphoribosyltransferase |
NK | = Natural Killer |
SVM | = Support Vector Machine |
UDP | = Uridine Diphosphate |
IHC | = Immunohistochemistry |
RNS-Seq | = RNA Sequencing |
ACKNOWLEDGEMENTS
Declared none.