All published articles of this journal are available on ScienceDirect.
Predicting Chronic Hyperplastic Candidiasis in the Tongue using Machine Learning: A Study of 186 Cases
Abstract
Introduction
This study examines the distribution of 186 Chronic Hyperplastic Candidiasis (CHC) cases verified by biopsy within the oral cavity, focusing on the prevalence in the tongue (72 cases) versus other oral locations (114 cases).
Methods
Utilizing the Random Forest Regressor (RFR), a robust machine learning algorithm, we analyze 16 unique risk factors to predict CHC incidence in the tongue. Linear regression is employed to evaluate the model's performance.
Results
The RFR demonstrates high accuracy in predicting CHC presence in various oral sites. The study highlights the impact of risk factors on CHC prevalence and the importance of CHC's location in the oral cavity for tailored diagnostic and treatment approaches. The findings suggest the Random Forest Regressor's potential as a tool for healthcare professionals in the early identification and diagnosis of CHC, enhancing disease understanding and improving patient care.
Conclusion
The RFR proves effective in predicting CHC occurrence in different oral areas. The clinical significance of Machine Learning method usage lies in the optimal evaluation of true pathogenetic factors and their relation patterns for CHC development in the tongue. Notably, most tongue CHC patients were non-smokers (63.9%), and female patients slightly outnumbered males (54.2%), challenging the common association of CHC with male smokers. A significant association exists between gastroesophageal reflux and tongue CHC (p=0.01), and a similar trend is noted for thyropathy in lingual lesions compared to other CHC locations (p=0.09). These findings underscore the necessity for clinicians to consider negative cultivations in lingual CHC cases (20.8% of cases), ensuring comprehensive evaluation and treatment.
1. INTRODUCTION
Chronic Hyperplastic Candidiasis (CHC) is a rare fungal infection affecting the oral cavity, characterized by persistent white or white-red lesions that fail to respond to conventional antifungal treatments [1-5]. This condition, also historically known as Candidal leukoplakia or Candi-dal epithelial hyperplasia, primarily arises from the over-growth of the opportunistic pathogen Candida albicans on the oral mucosa, and in superficial epithelium [6]. CHC represents a significant clinical challenge due to its chro-nic nature and persistent potential for oral squamous cell carcinoma development if left untreated. Oral epithelial dysplasia is frequent in CHC cases, so this disease is still a risky one, even if not a part of the oral potentially malignant disorders (OPMD) group. The actual 5th edition of OPMD 2022 does not contain CHC because of a lack of strong enough studies proving its precancerous potential [7]. Understanding the distribution of CHC in various locations within the oral cavity is essential for early detection, accurate diagnosis, and effective management [8-10].
The integration of machine learning methods into the complex landscape of healthcare systems and medical diagnosis has emerged as a transformative and highly influential force, delivering a multitude of substantial benefits to both patients and healthcare professionals [11-13]. In the current era, marked by an overwhelming abundance of data and unprecedented technological advancements, the significance of harnessing the potent capabilities inherent in machine learning cannot be overstated [14-16]. At the forefront of the merits attri-buted to machine learning in healthcare lies its exceptional capacity to discern intricate patterns within vast and multifaceted datasets. This aptitude for pattern recognition proves to be a paramount advantage, parti-cularly within the realm of medical diagnosis, where information can be overwhelmingly intricate and multi-farious. Machine learning algorithms demonstrate an aptitude for identifying subtle correlations and relation-ships that may elude human cognition [17]. These algorithms meticulously sift through an extensive array of data sources, encompassing electronic health records, medical imaging, genetic data, and beyond, to unveil concealed insights [18,19]. By doing so, they facilitate the early detection of disease, enabling timely interventions that can significantly augment patient outcomes [20]. This capability is of paramount importance in conditions where early diagnosis and prompt intervention can be genuine life-saving, such as cancer or cardiovascular diseases. Furthermore, machine learning serves as a harbinger of personalized medicine, bringing us closer to the reali-zation of tailored treatment plans that are meticulously designed to align with the unique requirements of each patient. By leveraging patient-specific data, including genetic profiles, medical history, and lifestyle variables, these algorithms empower healthcare providers to craft treatment strategies that are precisely aligned with the unique needs of each patient [21,22]. This level of personalization not only maximizes the efficacy of treatment but also minimizes the occurrence of adverse effects, thereby paving the way for more precise, indivi-dual-centric, and patient-focused healthcare.
The advent of machine learning in healthcare repre-sents a pivotal shift, offering unprecedented benefits by enhancing diagnostic accuracy, personalizing treatment, and improving patient outcomes. Leveraging vast datasets, these algorithms excel in identifying patterns and correlations beyond human discernment, crucial for intricate medical diagnostics. Machine learning's role extends to facilitating early disease detection and fostering the development of personalized medicine, tailored to individual patient profiles. This integration into healthcare systems signifies a move towards more precise, efficient, and patient-centered care, underscoring the transformative potential of machine learning in navigating the complexities of modern medical practice and research.
Recent advances in machine learning have revolu-tionized the field of medical research and have shown great promise in the early diagnosis and prognosis of various diseases [11,12, 20]. The integration of machine learning algorithms in medical data analysis offers a powerful tool to uncover intricate patterns and relationships, providing valuable insights into disease etiology and progression. Leveraging this technology, we aim to explore the distribution of CHC in the oral cavity, with a specific focus on the tongue, compared to other locations, and to develop a predictive model for CHC occurrence based on identified risk factors. This research endeavors to address several critical objectives:
We seek to comprehensively examine the distribution of CHC lesions in different locations within the oral cavity. While CHC predominantly affects the tongue, there may be variations in its occurrence in other intraoral sites, which are crucial for understanding its pathogenesis.
To build a robust predictive model, we identify and evaluate various risk factors associated with CHC. These factors include demographic characteristics, medical history, lifestyle habits, oral hygiene practices, and other potential variables that might influence the prevalence of CHC in different oral sites.
We employ the Random Forest Regressor (RFR), a powerful ensemble learning algorithm, as the machine learning method of choice for our predictive model. The RFR demonstrates exceptional performance in regression tasks, making it an ideal candidate for predicting CHC occurrence based on the identified risk factors.
Ensuring the reliability and accuracy of our predictive model is paramount. We subject the model to rigorous validation procedures using appropriate performance metrics, such as mean squared error, R-squared, and cross-validation techniques.
By shedding light on the distribution and predictive factors of CHC, our study provides valuable insights for clinical practitioners. Early detection and prompt inter-vention in high-risk individuals can significantly improve patient outcomes and reduce the burden of chronic oral infection.
Recent advances in machine learning have revolu-tionized the field of medical research, showcasing signi-ficant promise in the early diagnosis and prognosis of various diseases, including Chronic Hyperplastic Candi-diasis (CHC). The integration of machine learning algo-rithms into medical data analysis offers a potent tool for unveiling intricate patterns and relationships, thereby providing invaluable insights into disease etiology and progression.
2. MOTIVATION AND RESEARCH GAP
The significance of the proposed research in employing machine learning to predict CHC within the oral cavity, particularly in the tongue, is profound and multifaceted. By utilizing the Random Forest Regressor (RFR) to analyze 16 diverse risk factors, the proposed work represents a critical advancement in oral healthcare. The precision of the RFR in forecasting the presence of CHC provides healthcare providers with a potent tool for early detection and diagnosis. Importantly, the proposed study sheds light on specific risk factors and locations most susceptible to CHC, facilitating the development of customized diagnostic and treatment plans. This precision medicine approach accelerates timely and effective treatments and enhances patient care by addressing the unique aspects of each case. Moreover, the insights from the proposed research emphasize the importance of location in the prevalence of CHC, advocating for location-specific healthcare protocols. The integration of machine learning, especially the RFR, into predicting CHC cases signifies a significant leap in diagnosing and managing oral diseases, paving the way for advanced predictive models in clinical practice. This is expected to improve early detection rates significantly and contribute positively to public health outcomes.
The proposed study extends beyond identifying pathogenetic factors to provide a comprehensive view of their interplay, a task that is almost impossible to accomplish in real-time clinical settings. Leveraging advanced machine learning technology, it aims to map the prevalence of CHC across different oral locations and develop a predictive model based on a thorough analysis of identified risk factors. The choice of the RFR, renowned for its regression task performance, ensures the predictive model's reliability and accuracy. Hence, the proposed research offers crucial insights for clinical practitioners, enabling early intervention in high-risk individuals and markedly improving patient outcomes while addressing the challenge of this chronic oral condition effectively.
Figs. (1 and 2) illustrate two sample cases of patients who have experienced CHC in the tongue. These figures visually represent the presence and distribution of CHC lesions within the tongue for these two patients. However, it is important to note that while these figures show different patterns of CHC within the tongue, this research primarily considers the entire tongue as a single location when analyzing CHC distribution. In other words, the study treats the entire tongue as a single unit and does not investigate variations in CHC patterns across different regions or sections of the tongue.
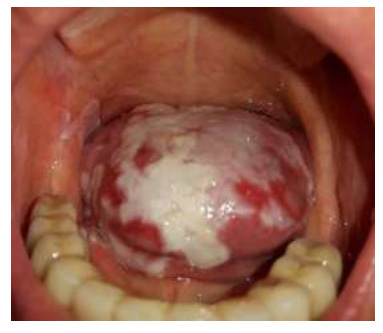
Visual representation of CHC in a patient's tongue, highlighting a distinct pattern of lesion distribution within the tongue tissue.
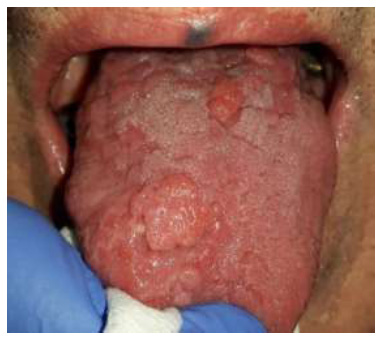
Depiction of CHC lesions in another patient's tongue, demonstrating a different pattern of CHC distribution and a different clinical hyperplastic presentation compared to Fig. (1).
The significance of this limitation is that it may overlook potential variations in CHC occurrence within the tongue, which could be crucial for understanding the disease's behavior and for tailoring diagnostic and treatment approaches. These figures serve as a visual representation of the need for future research to delve deeper into the location-specific patterns of CHC within the tongue, as such variations might have clinical implications for healthcare practitioners.
Predicting the location of CHC, especially on the tongue of a patient, is crucial for several reasons, and the findings from such predictions can be immensely helpful as guidelines for healthcare professionals:
Identifying the specific location of CHC, such as on the tongue, enables healthcare providers to diagnose the condition early. Timely diagnosis is essential for initiating appropriate treatment, as CHC can lead to discomfort, pain, and severe complications (oral epithelial dysplasia development) if left untreated. Accurate location prediction ensures that the treatment can be targeted to the affected area swiftly.
Different locations of CHC may require different treatment approaches. For instance, CHC on the tongue might require specific topical antifungal agents, adjustment to oral hygiene practices, and management of different induction factors than those present in the etiology of other locations of CHC lesions. Predicting the location and improving understanding of the disease can help physicians tailor treatment plans to the individual patient's needs, optimizing the chances of successful management.
Knowing the likely location of CHC can also guide preventive measures. Patients who are at higher risk for CHC on the tongue can be educated about specific oral hygiene practices (reducing a lingual coating), lifestyle modifications, medication side effects, or interventions that may reduce their susceptibility.
Improvement of CHC knowledge aids in resource allocation within healthcare facilities by optimization of dispensaries and by cooperation with immunologists and dermatologists.
Location-specific data on CHC can be valuable for epidemiological studies. Researchers can use this information to better understand the prevalence and patterns of CHC in different regions and populations. It can also provide insights into potential environmental or lifestyle factors contributing to the condition's occurrence in specific locations.
Accurate predictions regarding the location of CHC can enhance the overall patient experience. Patients can receive more targeted care and information about their condition, reducing anxiety and uncertainty.
By predicting the location of CHC, healthcare systems can work towards cost-effective management. Preventing severe cases or complications through early intervention can lead to cost savings in the long run by reducing the need for extensive treatment.
The application providing prediction of CHC occurrence by anamnestic data is very helpful to the clinician to pinpoint precise induction factors for an individual patient.
CHC, in the past also referred to as candidal leukoplakia, represents an enduring and localized manifestation of oral candidiasis, a fungal infection initiated by Candida species, particularly Candida albicans [23-25]. Candida, a form of yeast innately present in the human body, including the oral cavity, can undergo excessive proliferation and lead to infection under specific circumstances. CHC impacts the oral mucosa, the soft tissue lining within the mouth. It is distinguished by the emergence of non-removable, raised, and white or white-red patches or plaques on the mucous membranes. These patches typically lack pain and can manifest on various oral surfaces, including the tongue, buccal mucosa (interior lining of the cheeks), retro-commissural mucosa, palate (roof of the mouth), and other areas. Despite an incomplete comprehension of the precise origins of CHC, multiple predisposing elements are recognized as contributors to its emergence:
Individuals with compromised immune systems, such as those afflicted by Human Immunodeficiency Virus/Acquired Immune Deficiency Syndrome (HIV/AIDS), receiving chemotherapy, or undergoing organ trans-plantation necessitating immunosuppressive medications, exhibit heightened vulnerability to fungal infections like CHC.
Suboptimal oral cleanliness sets the stage for fungal overgrowth. Candida can thrive in environments laden with food remnants, plaques, and other factors that foster an imbalance in oral bacteria.
Individuals who utilize dentures face an escalated risk due to the potential accumulation of candidal micro-organisms in the denture material, coupled with the moist and anaerobic conditions beneath the dentures inducing decreased oral cavity pH.
Smoking and heavy alcohol consumption induce changes in the oral environment that favor the proliferation of Candida such as epithelial dystrophy and decreased pH in the oral cavity [26,27].
Malnutrition, particularly deficiencies in vital vitamins and minerals, can compromise the immune system's capacity to regulate the expansion of Candida. The diagnosis of CHC entails clinical scrutiny of oral lesions, supplemented by microscopic observation or culturing of tissue samples to validate the presence of Candida species [28]. The treatment predominantly encompasses anti-fungal interventions, such as the application of topical antifungal gels and creams, oral antifungal medications, or, in select cases, antifungal mouthwashes [29-32]. Neglecting to address the underlying predisposing factors can culminate in CHC, evolving into a persistent ailment, with the potential for lesions to resurface even following treatment. To avert this outcome, regular dental examinations, diligent adherence to oral hygiene routines, and management of underlying medical conditions stand as imperative measures.
To encapsulate, CHC denotes a persistent fungal infection influencing the oral mucosa, marked by raised and white patches. Although Candida overgrowth is a central instigator, several elements like immuno-deficiency, immunosuppression, medication side effects [33,34], concomitant disease complications [35-38], suboptimal oral hygiene, denture use, and lifestyle choices (cigarette smoking and alcohol consumption) can contribute to its inception. Appropriate diagnosis, treat-ment, and mitigation of predisposing factors emerge as pivotal facets in effectively managing CHC.
3. METHODOLOGY
3.1. Inclusion and Exclusion Criteria
In pursuit of our study's goals, we utilized a retrospective analysis based on the records of 186 cases of Chronic Hyperplastic Candidiasis (CHC) confirmed through biopsy and treated at the University Hospital Pilsen, Faculty of Medicine, Charles University, from 1995 to 2023. We gathered data concerning their demo-graphics, medical history, clinical features, and estab-lished risk factors. The study specifically targeted participants with clinical lesions marked by hyperplasia (an increase in cell numbers causing the tissue to enlarge) and hyperkeratosis (the thickening of the skin or mucous membrane's outer layer), which are akin to leukoplakia (white patches) or leukoerythroplakia (white and red patches). These conditions are commonly observed in the oral cavity and may be confused with one another due to their visual resemblance. Nonetheless, for a lesion to be included in this study as CHC, the lesion needed to be confirmed via biopsy-a process in which a small tissue sample is extracted for detailed microscopic examination. A key aspect of our study's methodology involved the rigorous examination of biopsy samples, which were required to exhibit positive staining for candidal hyphae upon application of the Periodic Acid Schiff (PAS) staining technique. PAS staining is a specialized histochemical method employed to accentuate fungal organisms within tissue samples, making it an invaluable tool in the diagnosis of fungal infections, including CHC. This technique works by staining polysaccharides and fungal cell wall components, resulting in a distinctive magenta coloration of the fungal elements. Such clear, vivid staining allows for the unequivocal identification of Candida species, the primary fungal pathogen implicated in CHC, under microscopic examination. The effectiveness of PAS staining in revealing the presence of Candida species is paramount for our study, as it directly influences the accuracy of CHC diagnosis.
By meticulously ensuring that all included biopsy samples exhibited positive PAS staining for candidal hyphae, our study upheld a high standard of diagnostic accuracy. This rigorous methodology not only bolsters the validity of our findings but also highlights the necessity of using dependable diagnostic criteria in research on the prevalence and nature of fungal infections in the oral cavity. Our careful approach aids in deepening the understanding of Chronic Hyperplastic Candidiasis (CHC), paving the way for progress in its diagnosis and treatment. To enhance the study's specificity and reliability further, we meticulously excluded any oral cavity lesions that, while clinically suggestive of CHC, did not have their diagnosis confirmed through biopsy evidence.
This stringent exclusion criterion was carefully chosen to ensure the incorporation of only those cases presenting definitive histopathological evidence of CHC. Such a methodological decision was pivotal in sharpening the focus of our research, centering it firmly on instances of CHC that could be unequivocally verified through histological analysis. This approach not only reinforces the integrity and specificity of our findings but also minimizes the risk of data contamination by potential misdiagnoses or ambiguous cases. By adopting this rigorous selection process, our study contributes valuable, high-precision data to the existing body of knowledge, offering a clearer, more accurate picture of CHC's epidemiology, pathology, and potential therapeutic targets. This enhancement in data quality is essential for advancing the scientific community's understanding of CHC and lays a solid foundation for future research aimed at developing more effective diagnostic techniques and treatment protocols for this condition.
3.2. The Proposed ML Methods
In our methodology, we employed two pivotal machine learning (ML) approaches to meticulously analyze and interpret our dataset: Linear Regression Machine and Random Forest Regressor. These methods were integral in our comprehensive examination of CHC occurrence across various oral locations and in identifying potential risk factors associated with the disease. Our dataset comprised detailed information on diseases such as arterial hypertension, bronchial asthma, diabetes mellitus, gastroesophageal reflux, and thyropathy, alongside medication usage (antihypertensives, local and systemic corticosteroids), daily fluid intake, and cigarette smoking habits. We categorized cigarette smoking volumes into non-smoking, smoking up to 10 cigarettes a day, and smoking more than 10 cigarettes a day. Additionally, we conducted the Volumetric Skach test to measure both unstimulated and stimulated salivation for 15 minutes each, followed by saliva pH testing albicans and non-albicans Candida, with cultivation values graded from negative to 150 CFU.
Before analysis, we preprocessed the dataset to manage missing values, and outliers, and normalize the data. We then performed a descriptive analysis to under-stand the distribution of CHC in different oral locations, employing frequency distributions and visualizations for a comprehensive overview. To unearth potential risk factors associated with CHC occurrence, we embarked on both univariate and multivariate analyses. These included the use of chi-square tests, t-tests, and logistic regression, providing a statistical assessment of each identified risk factor's significance. Building upon this foundation, we deployed the Random Forest Regressor to craft a predic-tive model for CHC occurrence, training it on the elucidated risk factors. Validation was conducted through k-fold cross-validation, ensuring the model's robustness and generalizability [39-42].
The Random Forest Regressor algorithm creates a “forest” of decision trees, each generated from random subsets of training data and features to prevent overfitting-where models perform well on training data but poorly on new data. After training, it combines the trees' predictions, usually averaging them for regression tasks, to produce a final, more accurate output. This method's ensemble nature makes it robust against noisy data and outliers, enhancing its predictive accuracy and ability to generalize to unseen data. It is adept at uncovering the importance of different features and capturing complex, non-linear relationships within the data. Hyperparameter tuning, such as adjusting the number of trees, their maximum depth, and the features used for splitting, is crucial for optimal performance. Despite its computational demands, parallel processing capabilities help manage its scalability. The Random Forest Regressor is valued for its versatility and effectiveness in predicting numeric outcomes, balancing the ensemble's diversity against overfitting risks, with careful data preparation and hyperparameter adjustment essential for achieving its full potential.
In the proposed method, we adopted a standard practice in machine learning by dividing our dataset into training and testing sets to evaluate our models effectively. Specifically, 70% of the data was allocated for training both the linear regression machine and the random forest regressor. This allocation allows the models to adequately learn the relationship between the input features and the target variable. The remaining 30% of the data was set aside for testing, serving as a novel dataset to assess the models' predictive capabilities. This segmentation is critical, as it ensures the models' performances are evaluated on new data, revealing their accuracy and ability to apply learned patterns to unseen data. Such a split is crucial for verifying that the models are truly learning and not merely memorizing the training data, thus demonstrating their applicability in real-world scenarios.
4. RESULTS
Figs. (3 and 4) illustrate how we used the Linear Regression method to estimate the likelihood of CHC occurring specifically on the tongue as opposed to other areas within the mouth, using training data for the analysis. Here's a breakdown of what each part of these figures represents for a clearer understanding [43-45].
Y-axis (Vertical): This axis categorizes different parts of the oral cavity into two main groups: the tongue (TG) and other locations (OL) within the mouth. This classification helps us examine and compare the occurrence of CHC in these distinct areas.
X-axis (Horizontal): This axis shows the spread or distribution of patients within the study, essentially randomizing their arrangement to avoid any bias in the analysis.
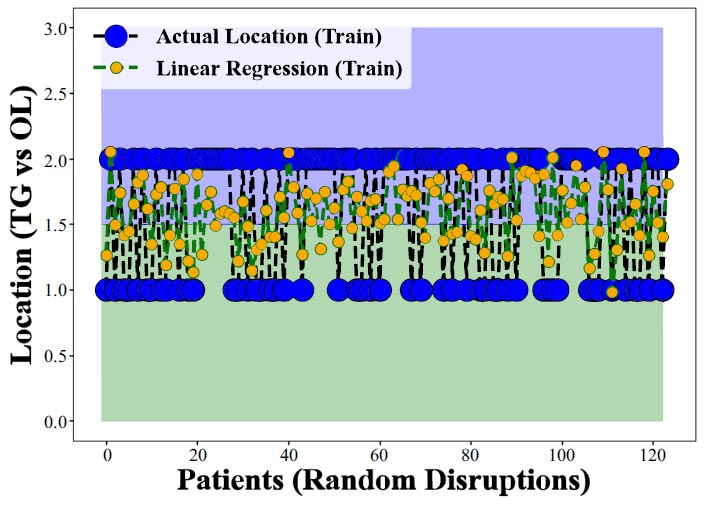
Performance assessment of linear regression in predicting CHC occurrence across various oral locations. notably, the model struggles to distinguish CHC presence in the tongue from other sites (training data).
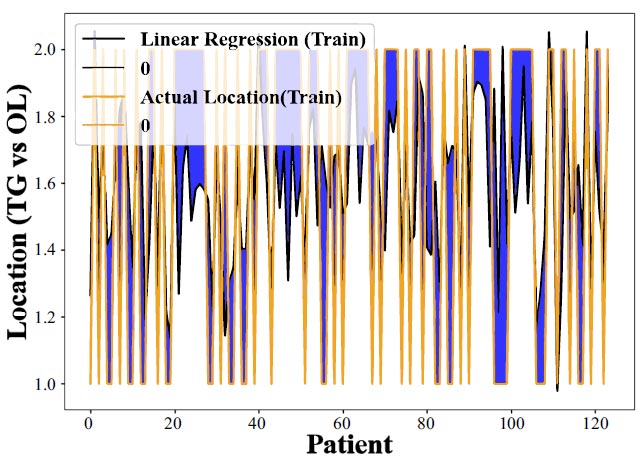
Further evaluation of the linear regression model's predictive capabilities for CHC occurrence in different oral locations, revealing its limited success. This emphasizes the need for more advanced predictive methods to enhance location-specific CHC forecasting accuracy (training data).
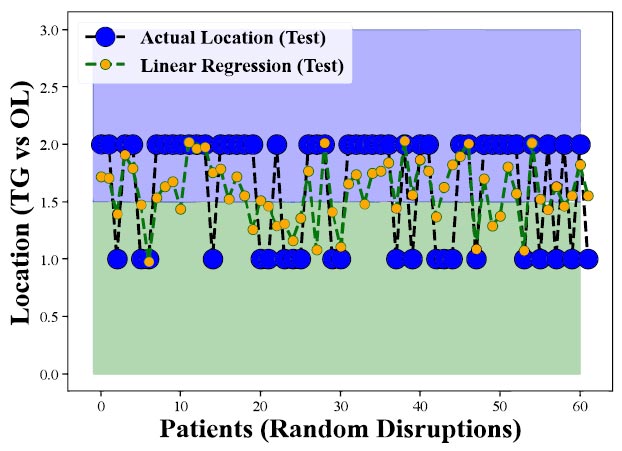
Performance of Linear Regression on Test Data demonstrates the model's worse results during testing, showcasing its limited ability to predict CHC occurrence in the tongue and other oral locations.
Accuracy or Predictive Performance: The effectiveness of the Linear Regression model in predicting CHC's occurrence in these areas is measured by comparing the actual clinical findings with the model's predictions. Essentially, the closer the model's output is to the actual clinical measurements, the more accurate or successful the model is considered.
The primary goal here was to see if the Linear Regression model could more accurately predict CHC's occurrence on the tongue compared to other areas in the mouth. Ideally, a successful model would demonstrate higher accuracy for tongue predictions, indicating it could specifically identify CHC cases in this location effectively.
However, the findings from Figs. (3 and 4) reveal that the Linear Regression model does not perform well in predicting CHC on the tongue versus other locations, showing lower accuracy or effectiveness for tongue-related CHC cases. This suggests that the model has difficulty distinguishing between CHC occurrences on the tongue and in other oral cavity parts. The results highlight the limitations of using Linear Regression for such specific predictions and the potential need for more complex models, like the Random Forest Regressor, to enhance prediction accuracy for CHC in different oral locations.
Figs. (5 and 6) further explore the model's perfor-mance but focus on test data-data not) used during the model's training phase. These figures are crucial because they assess how well the model can apply what it learned during training to new, unseen data. The observed decrease in performance when applying the model to test data indicates challenges in the model's ability to generalize its predictions to new cases, underscoring the ongoing need to refine the model or consider alternative methods for better predictive accuracy in diagnosing CHC.
Figs. (7 and 8) display the outcomes of applying the Random Forest Regression technique to anticipate the presence of CHC on the tongue in comparison to other oral locations (utilizing training data). In these figures, the y-axis denotes various oral sites, including the tongue (TG), and other locations (OL), while the x-axis represents the random patient distribution. The model's accuracy or predictive capability for each location is depicted by the disparity between the actual clinical measurements and the model's output. These figures illustrate the Random Forest Regression method's efficacy in forecasting CHC occurrence across diverse oral sites. Generally, if the model were highly proficient, we would anticipate observing higher accuracy values for the tongue compared to other sites, signifying the model's superior performance in predicting CHC specifically within the tongue. However, it becomes apparent from both (Figs. 7 and 8) that the Random Forest Regression's predictions are successful in accurately foretelling CHC occurrence in the tongue compared to other locations. The lower accuracy or weaker performance for the tongue suggests that the model faces challenges in distinguishing between CHC
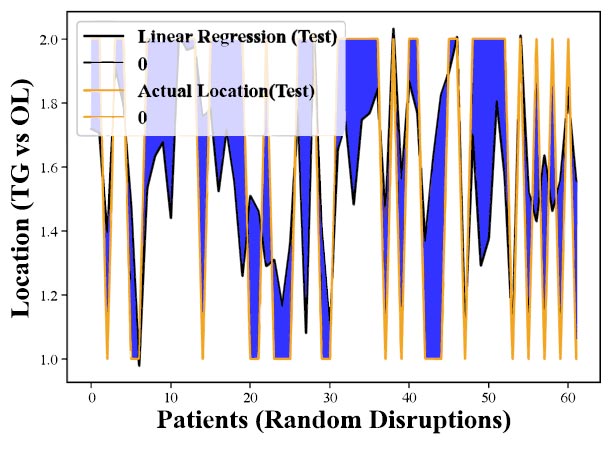
Assessment of Linear Regression on Test Data indicates a notable decline in predictive performance when applied to previously unseen cases, emphasizing the need for model refinement to enhance its reliability in real-world scenarios.
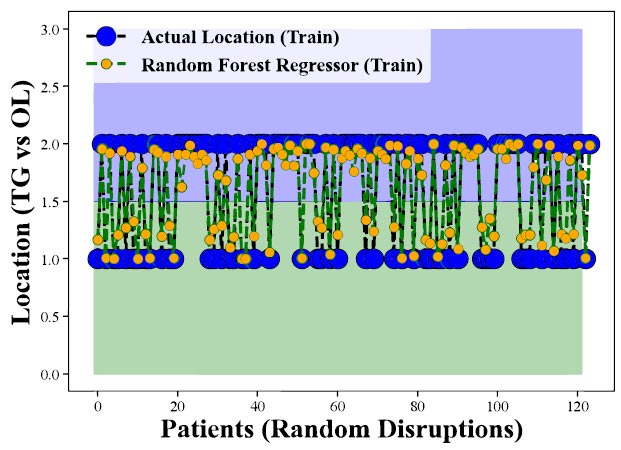
Evaluation of Random Forest Regression in Predicting CHC Occurrence Across Oral Locations (Training Data). Highlights the model's performance discrepancies among oral sites, emphasizing its effectiveness in forecasting CHC presence in the tongue.
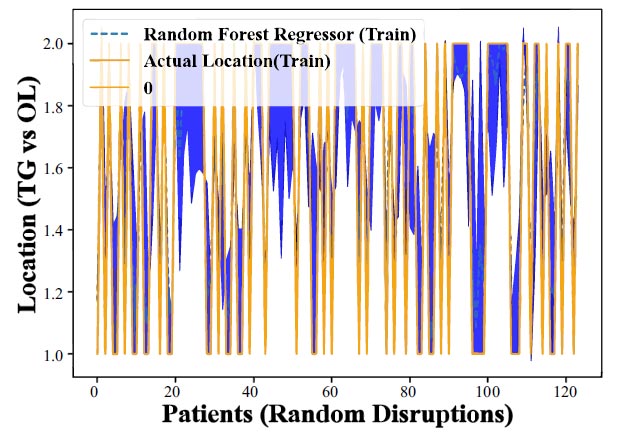
Assessing Random Forest Regression's Predictive Power for CHC Occurrence Across Oral Locations (Training Data). Reveals the model's varying success rates in predicting CHC in different oral sites, showcasing its ability to effectively identify CHC cases in the tongue.
cases in the tongue and those occurring in other parts of the oral cavity. Despite this, the model's success in prediction underscores the value of employing the Random Forest Regression method.
Fig. (9) represents the results of applying the Random Forest Regression method, specifically with test data, to predict the occurrence of CHC on the tongue compared to other locations within the oral cavity. Similar to (Figs. 7 and 8), the y-axis is expected to denote various oral sites, such as the tongue (TG), and other locations (OL), while the x-axis may represent random patient distribution. The accuracy or predictive performance of the model for each location is likely indicated by the differences between the actual clinical measurements and the model's output. This figure illustrates how well the Random Forest Regression method performs when applied to test data, evaluating its ability to predict CHC occurrence across different oral locations. A successful model should exhibit higher accuracy values for the tongue compared to other locations, indicating its proficiency in forecasting CHC specifically within the tongue.
Upon examining Figs. (9), we can consider the applicability of the Random Forest Regression method in predicting CHC in a real-world context, using data that the model has not been trained on. The outcomes from this test data can provide insights into the model's generalization capacity and its suitability for practical use. These findings strongly suggest a significant relationship between the identified risk factors and the occurrence of CHC, particularly in the tongue. The model's successful predictions for CHC on the tongue, even when faced with new and unseen data, underscore the robustness of the identified risk factors in influencing the presence of CHC. This highlights the potential utility of these risk factors in clinical practice for early identification of CHC cases in the tongue, ultimately benefitting patient care and public health.
The comparison of tongue cases and those of other locations on the incidence of gastroesophageal reflux disease (GERD) and thyropathy, the volume of cigarette smoking, and corticosteroid usage is shown in Table 1.
A comparison of unstimulated and stimulated saliva-tion from the Skach test, pH of saliva (both unstimulated and stimulated), and colony forming units (CFU) of C. albicans and non-albicans Candida means between the tongue and other location cases is shown in Table 2.
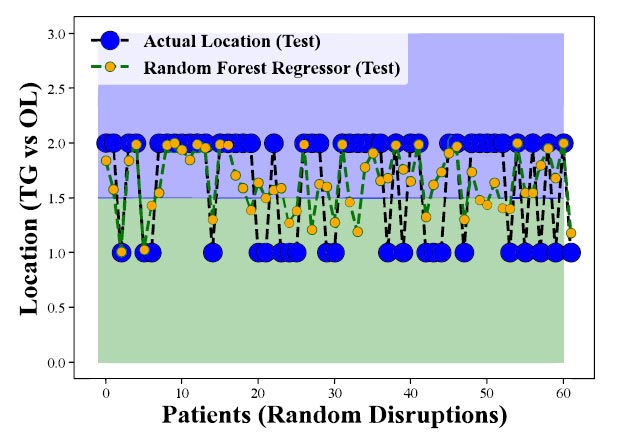
Evaluation of Random Forest Regression's Predictive Performance for CHC Occurrence Across Oral Locations (Test Data) demonstrates the model's effectiveness in forecasting CHC presence in the tongue and other sites, affirming the strong relationship between risk factors and CHC occurrence.
CHC Cases | 186 Male:female Ratio 1:1,07 (N=90 Male, 96 Female) |
---|---|
Tongue Cases: Other Locations |
Tongue 38,7% (n=72), Other locations 61,3% (n=114) |
Gastroesophageal Reflux |
Tongue 41,7% (n=30), Other locations 19,3% (n=22) |
Thyropathy | Tongue 25% (n=18), Other locations 14,9% (n=17) |
Sex in Tongue Cases | Males 45,8% (n=33), Females 54,2% (n=39) |
Nicotinism in tongue Cases | Non-smoking 63,9% (n=46), Smoking to 10/day 19,4% (n=14), Smoking +10/day 16,7%(n=12) |
Costicosteroid Usage | Systemic corticosteroids 12,5% (n=9) |
In Tongue Cases | Local corticosteroids 48,6% (n=35) |
- | Tongue Mean | Other Locations Mean |
---|---|---|
Salivation Unstimulated |
3,67 ml/15 min |
4,01 ml/15 min |
Salivation Stimulated |
15,45 ml/15 min |
17,02 ml/15 min |
PH Unstimulated Saliva |
6,39 | 6,14 |
PH Stimulated Saliva |
7,14 | 7,0 |
C. Albicans CFU | 40,8 | 42,46 |
C. Non-albicans CFU | 2,2 | 8,1 |
In our retrospective study on CHC, we observed a notable predominance of female patients, particularly for lesions located on the tongue, where they represented 54.2% of cases. This contrasts with the prevalent association of CHC with male smokers, especially since lesions on the tongue were primarily found in non- smokers, accounting for 63.9% of cases. Furthermore, a significant difference was identified in the habit of smoking more than 10 cigarettes a day, with a higher incidence in non-tongue locations (p=0.001). Crucial risk factors for CHC, especially in tongue lesions, include gastroesophageal reflux disease (GERD), which showed a stronger association (p=0.01) compared to other locations. Thyropathy's prevalence in tongue CHC cases (p=0.09) further highlights the unique etiological factors in this site. Another critical observation was the low yield of C. albicans and non-albicans Candida CFU in tongue CHC, with 20.8% of cases displaying repeatedly negative cultivation from mucosal swabs, emphasizing the rarity of non-albicans Candida association in lingual CHC. These findings underscore the need for vigilant diagnostic practices due to the distinctive risk profile and etiology of CHC in the tongue, including the significant roles of GERD and thyropathy.
5. DISCUSSION
Accurate prediction of its occurrence can greatly assist physicians not just in timely diagnosis but specifically in the treatment and management of an individual regimen for patients. In this study, we explore the effectiveness of a predictive model, specifically Random Forest Regression (RFR), in forecasting the occurrence of CHC on the tongue. Our findings indicate that this method demonstrates promising results and can serve as a valuable guideline for physicians in analyzing and managing this condition. Using RFR, the clinician can verify the considered induction factors associated with CHC development to improve individual therapy of patients. The way RFR is able to understand the presented anamnestic and clinical data gives us important dimensions of factorial interaction, which can otherwise stay hidden. Before delving into our findings, it is crucial to understand Chronic Hyperplastic Candidiasis. This condition is primarily characterized by the persistent presence of candidal hyphae in the tongue's superficial mucosa [39-42]. It often appears as white, thickened plaques or patches, leading to discomfort and potential complications if left untreated [39-42]. Improvement of this chronic disease management allows for better resource allocation within healthcare facilities.
The analysis revealed that the Random Forest Regression model achieved a high level of accuracy in predicting the occurrence of CHC on the tongue. The model's ability to consider multiple variables simultaneously made it well-suited for this task. Through feature importance analysis, we used several key risk factors associated with the development of CHC. The proposed model also exhibited the ability to identify trends in the occurrence of candidiasis on the tongue. One of the significant advantages of our predictive model is its potential for personalized medicine. By considering individual patient profiles, including their medical history and risk factors, physicians can tailor prevention and treatment plans to each patient's unique needs. The findings from our study have several important implications for clinical practice: Physicians can use the predictive model to identify patients at higher risk of developing CHC. This enables early intervention and treatment, potentially preventing the condition from worsening.
Healthcare facilities can then optimize the resource allocation of dispensaries. This ensures that sufficient resources, such as medications and healthcare personnel, are available when needed. Armed with the knowledge of risk factors, healthcare providers can educate patients about preventive measures, such as maintaining good oral hygiene practices and managing underlying medical conditions. The study opens avenues for further research into the prevention and treatment of Chronic Hyperplastic Candidiasis. By understanding the key risk factors, researchers can explore new therapies and interventions to reduce the incidence of this condition. The findings of this research shed light on the distribution and risk factors associated with CHC in various oral locations. The clinical implication of the study is significant, as proper treatment can prevent the progression of CHC and reduce the potential for oral epithelial dysplasia development within CHC lesions with its risk of malignant transformation [39-42]. Additionally, a better understanding of the risk factors contributing to CHC occurrence could aid in the development of targeted prevention strategies and personalized treatment approaches. These personalized approaches are designed to enhance the likelihood of treatment efficacy, thereby translating to improved outcomes and greater patient satisfaction.
CHC in tongue location can be risky for clinical differentiation due to frequent negative Candida cultivation. CHC in general is believed to be associated with male smokers [39-42], but our retrospective study revealed that for the development of lingual CHC lesions, different induction factors, as most of the patients (63,9%) were non-smokers and females slightly prevailed with 54,2%. Moreover, gastroesophageal reflux and thyropathy have been more frequent in tongue locations in comparison to other CHC locations.
The integration of machine learning techniques in the analysis of CHC distribution and prediction represents a cutting-edge approach in oral disease research. The results of this study have the potential to revolutionize clinical practice, offering clinicians a powerful tool to enhance patient care and improve overall oral health outcomes. By leveraging the power of machine learning, we contribute to the advancement of medical knowledge and improve the management of CHC. Exploring the application of machine learning, particularly through the utilization of the Random Forest Regressor predictive model, is aimed at enhancing our understanding, diagnosis, and management of CHC. This condition affects various regions within the oral cavity, prompting us to delve into ways in which machine learning can effectively address this challenge:
In the realm of medical investigation, the adeptness of machine learning algorithms in handling and dissecting vast datasets becomes particularly crucial [12,46].
The significance of this study is highlighted through a comparative analysis with existing research, focusing on the utilization of machine learning models, specifically the Random Forest Regressor, in identifying and predicting Chronic Hyperplastic Candidiasis (CHC) across various patient demographics. Unlike traditional statistical approaches, this model adeptly analyzes a wide range of patient-specific data, including demographic details, clinical symptoms, and potential risk factors, to uncover complex patterns and relationships that may not be readily discernible. This comprehensive analysis facilitates the early detection of CHC by predicting its likelihood in different patient groups, thereby offering a significant advantage over conventional methods. The predictive model's ability to provide early warnings to healthcare providers ensures prompt intervention and treatment, crucially preventing the progression to more severe conditions. This study underscores the transformative potential of machine learning in enhancing diagnostic processes and improving patient outcomes through early detection and prevention strategies.
The prowess of machine learning models also extends to the identification of subtle correlations between various risk factors and the initiation of CHC. This exploration unravels valuable insights into the fundamental triggers and progression of the ailment. Isolating specific risk determinants empowers the development of targeted prevention strategies, allowing medical professionals to channel their efforts toward mitigating the most influential factors. The integration of machine learning into clinical practice promises revolutionary
transformation. Traditional diagnostic and treatment methodologies might not encompass the intricate complexities associated with CHC occurrence and progression. Machine learning presents a supplementary tool that aids clinicians in delivering precise diagnoses, projecting outcomes, and shaping intervention strategies. This shift holds the potential for optimizing healthcare resource utilization and elevating patient outcomes [2, 27,28].
Continuous learning and enhancement are intrinsic to machine learning models. The accumulation of additional patient data allows the model to fine-tune its predictions and insights. This iterative process culminates in a predictive tool that becomes increasingly accurate and dependable over time, evolving in tandem with advances in medical knowledge.
In summation, the integration of machine learning methodologies, exemplified by the implementation of the Random Forest Regressor model, possesses the capacity to revolutionize oral disease research. Through the analysis of data patterns, projection of CHC occurrences, identification of risk factors, and customization of treatment strategies, machine learning empowers clinicians to make informed decisions, provide timely interventions, and ultimately elevate the standards of patient care and oral health outcomes.
This study's limitations include the categorization of Candida Colony forming units into discrete groups (e.g., negative, 5, 15, 30, 100, 150) rather than treating them as continuous variables, which could have enabled the acquisition of more precise values for evaluation. Furthermore, the clinical comparison of this study is challenging due to the significantly larger group of CHC cases examined and the criteria evaluated, which differ from those in other studies.
CONCLUSION
In this study, we investigated the distribution of Chronic Hyperplastic Candidiasis (CHC) in different locations in a study cohort of 186 CHC cases, with a particular focus on the tongue (n=72). Leveraging the Random Forest Regressor (RFR) as the machine learning method, we analyzed 16 identified risk factors to predict the occurrence of CHC. Additionally, linear regression was utilized to measure the model's performance.
Our results demonstrate that the RFR exhibits a remarkable capability in accurately predicting the incidence of CHC in various oral locations. The acceptance of this machine learning approach suggests its potential in supporting medical professionals for early detection and diagnosis of CHC, thereby enabling prompt and targeted interventions.
These insights contribute to a better understanding of the etiology and pathogenesis of CHC, potentially guiding future research efforts and treatment strategies. The clinical significance of Machine Learning method usage lies in the optimal evaluation of true pathogenetic factors and their relation patterns for CHC development in the tongue.
Overall, the integration of machine learning, specifically the Random Forest Regressor, into the analysis of CHC distribution in the oral cavity holds promise for advancing our understanding of this condition and improving patient care. As technology continues to evolve and data availability increases, the RFR's predictive power can be further enhanced, leading to more precise and personalized medical approaches in the field of oral health. We hope that our findings inspire further investigations and encourage the application of machine-learning techniques in oral disease research and clinical practice.
LIST OF ABBREVIATIONS
AIDS | = Acquired Immune Deficiency Syndrome |
CFU | = Colony Forming Unit |
CHC | = Chronic Hyperplastic Candidiasis |
GERD | = Gastroesophageal Reflux Disease |
HIV | = Human Immunodeficiency Virus |
OL | = Other Locations |
OPMD | = Oral Potentially Malignant Disorder |
LR | = Linear Regression |
ML | = Machine Learning |
PAS | = Periodic Acid Schiff |
TG | = Tongue |
ETHICAL STATEMENT
This project was approved by the Institutional Review Board (IRB) and EC of the University Hospital in Pilsen, with a positive opinion dated 03/09/2009. Helsinki declaration has been followed for the study.
AVAILABILITY OF DATA AND MATERIALS
The data supporting the findings of this study are derived from the University Hospital Pilsen, Faculty of Medicine in Pilsen, Charles University. The datasets used are available from the corresponding authors [O.M] upon reasonable request.